No one really likes risk. We all have to deal with it, but that doesn’t mean we want to. Yes, there are people who live to jump out of airplanes and walk on tight ropes with no net, but I’m talking about financial risk.
Unfortunately, at least in investing, risk and return are tightly intertwined. If you want to do better than just sticking your money in the bank, you have to accept some level of risk. But what if there was a better way? What if we could create trading strategies that would allow us to get better returns with less risk?
As we’ve seen, you can’t time the markets simply through guessing whether the stock market as a whole is going to do well or not. But what if we could be a little “smarter.”
As you can imagine, there’s no shortage of folks looking for ways to do this (see large swaths of the financial services industry and financial media). And a lot of people like to look at different valuation metrics as the answers.
Timing (Part of) the Markets
One of the common things that people seem to gravitate towards is trying to time the different risk premiums available in the markets.
So we wanted to see if there is a way to reliably predict what the risk premiums will do in the future using different valuation metrics. If that’s possible, we should be able to capture premiums when they look high and avoid them when they look low. This would pretty much be the holy grail of finance.
The only problem is, there’s no evidence that you can actually do this.
Wei Dai and Audrey Dong of Dimensional Fund Advisors did a study that tried to identify ways of predicting future risk premiums based on a number of different trading rules. They focused on four of the most prominent risk premiums in the stock market:
- The Equity Premium – the difference in returns between stocks and bonds
- The Size Premium – the difference in returns between small stocks and large stocks
- The Value Premium – the difference in returns between stocks with low price to book ratios (value stocks) and stocks with high price to book ratios (growth stocks)
- The Profitability Premium – the difference in returns between stocks with high relative profitability and stocks with low relative profitability.
They generated over 700 different strategies using relative valuation metrics, mean reversion, and momentum to identify when to be in or out of the different premiums – and tested them in the US, Developed ex US, and Emerging Markets.
Did They Work?
So how did those strategies work out?
Not particularly well.
Overall, the strategies underperformed simply staying invested in their respective premiums by 1.1% per year. That’s a big difference.
And these numbers don’t even consider the trading costs or taxes that you would incur with all of the trading that a timing strategy requires.
However, there were some rules that seemed to work – meaning that, from a statistical standpoint, there was a less than 5% chance that their performance was the result of chance. In fact, 30 out of the 720 strategies that met the criteria, or 4.2% of the strategies.
Which is suspiciously close to the 5% false positive rate that you would expect when you’re looking a 95% level of confidence. Which leads me to suspect that these rules aren’t something that you could use going forward.
Exceptions Prove the Rule
There was one interesting bright spot in the analysis (at least if you were looking to time the premiums). All 20 of the strategies that used momentum (which is essentially the idea that if you have good/bad returns in one period, you’ll likely have them again in the next period) had reliably positive returns. In fact, these 20 strategies averaged a 0.75% positive annual premium from July 1927 through December 2022.
However, when you take a look at the underlying data, it was really driven by a single two year period in the 70’s. Since the size premium was (really) negative in 1972, most of the momentum strategies would have been out of small cap stocks in 1973 – when the size premium had an annual return of -20.64%. This accounted for 0.33% of the 0.75% annual outperformance.
While this is absolutely part of the data, and something that is important to consider, it’s also important to note that this is definitely an outlier. When you look at only the second half of the data (from 1975-2022), none of these momentum strategies had reliable positive returns relative to simply staying invested.
Timing the Premiums in Practice
But what about the strategy that they were talking about with lots of charts and graphs on CNBC?
It’s impossible to prove a negative, and the markets are not perfectly efficient. So I can’t say that the strategy won’t work. But it seems pretty unlikely.
If you step back and think about the logic, it shouldn’t come as a surprise that we can’t time the premiums – at least with publicly available information. Financial information is everywhere, and everyone in the market is aware of it. Which means it’s already baked into the prices.
And if that information is already in the prices, then that information doesn’t give us any advantage. It doesn’t tell us what stocks are going to do next. Stocks move based on the next piece of information – and, specifically, how that next piece of information squares with what the markets expected. Until we can predict the future, we can’t predict how the market will move.
So What Should You Do?
Timing the premiums works just as well as timing the markets.
It doesn’t.
You can always find amazing strategies when you are looking at back testing, and seeing what worked in the past. But what matters is what will happen next. And it’s not looking promising that there are any timing strategies that you can use going forward.
Just like always, you want to plan for the long term. Decide what risks you want to include in both your retirement income plan and investment portfolio, and stick with it.
There simply is no magic trading strategy that will help you beat a boring “buy and hold” strategy. And trying to find one isn’t going to help you.
Like this article? Download our free eBook!
Our eBook The 9 Secrets of Intelligent Investors breaks down the guiding principles to help you make informed investment decisions.
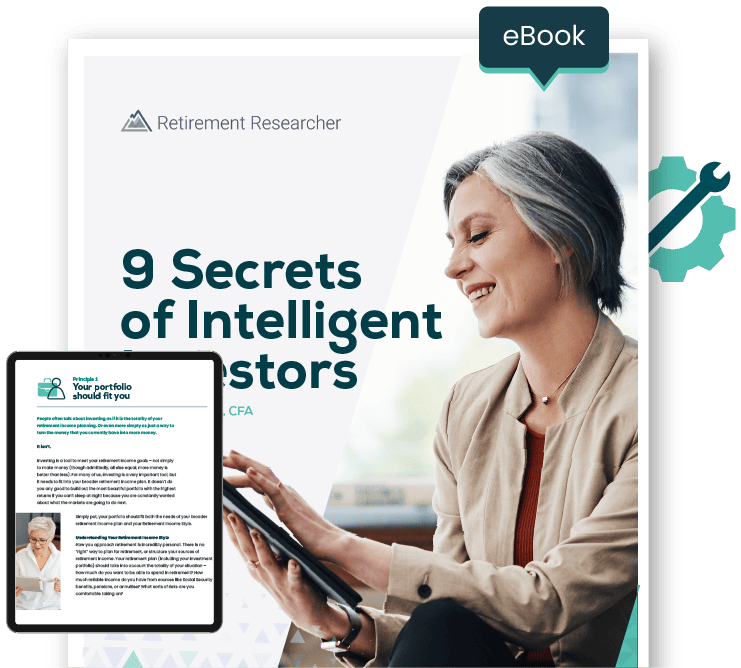